Although understanding machine learning lab requirements or getting your head around it isn’t always simple, particularly when you don’t have any prior knowledge of it, finding the right information of it is not as straightforward as you might think.
Not to worry though as the article below puts you out of the stress of fretting about machine learning lab requirements. All you’ve just got to do is read on to get the latest information on hardware and software requirements for machine learning projects, artificial intelligence lab requirements, hardware requirements for machine learning projects, and hardware for machine learning course. You can also find up to date information on computer hardware for machine learning in related articles on Collegelearners.
Machine learning is a subset of artificial intelligence function that provides the system with the ability to learn from data without being programmed explicitly.
Machine learning is basically a mathematical and probabilistic model which requires tons of computations. It is very trivial for humans to do those tasks, but computational machines can perform similar tasks very easily.
Consumer hardware may not be able to do extensive computations very quickly as a model may require to calculate and update millions of parameters in run-time for a single iterative model like deep neural networks.
Thus, there is a scope for the hardware which works well with extensive calculation. But before we dive deep into hardware for ML, let’s understand machine learning flow.
There are four steps for preparing a machine learning model:
- Preprocessing input data
- Training the deep learning model
- Storing the trained deep learning model
- Deployment of the model
Among all these, training the machine learning model is the most computationally intensive task.
Now if we talk about training the model, which generally requires a lot of computational power, the process could be frustrating if done without the right hardware. This intensive part of the neural network is made up of various matrix multiplications.
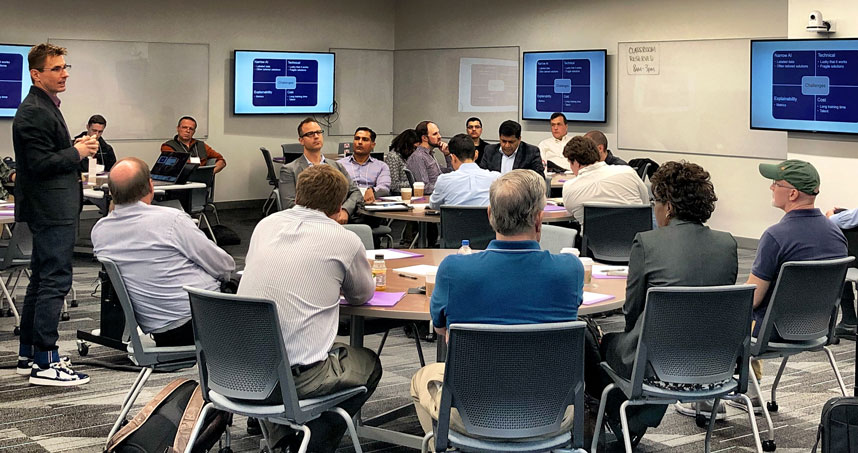
So how can we make the training model faster?
This can be accomplished simply by performing all the operations at the same time, instead of taking them one after the other. This is where the GPU comes into the picture, with several thousand cores designed to compute with almost 100% efficiency. Turns out these processors are suited to perform the computation of neural networks as well.
The fight between CPUs and GPUs favors the latter because of the large amount of cores of GPUs offsetting the 2–3x faster speed of CPU clocks – ~3500 (GPU) vs ~16 (CPU). The GPU cores are a streamlined version of the more complex CPU cores, but having so many of them enables GPUs to have a higher level of parallelism and thus better performance.
CPUs are designed to run almost any calculation, that is why they are called general-purpose computers. In order to achieve this generality, CPUs store values in registers, while a program tells the Arithmetic Logic Units (ALUs) which registers to read, perform an operation (such as an addition, multiplication or logical AND) and which register to use for output storage, which in turn contains lots of sequencing of these read/operate/write operations. Due to this support for generality (registers, ALUs and programmed control), CPUs cost more in terms of power and chip area.
There are alternatives to the GPUs such as FPGAs and ASIC, as all devices do not contain the amount of power required to run a GPU (~450W, including CPU and motherboard). TPU (Tensor Processing unit) is another example of machine learning specific ASIC, which is designed to accelerate computation of linear algebra and specializes in performing fast and bulky matrix multiplications.
TPU
Google Search, Street View, Google Photos, and Google Translate, they all have something in common – Google’s accelerated neural network also known as TPU. It is one of the most advanced deep learning training platforms. TPU delivers 15-30x performance boost over the contemporary CPUs and GPUs and with 30-80x higher performance-per-watt ratio. The TPU is a 28nm, 700MHz ASIC that fits into SATA hard disk slot and is connected to its host via a PCIe Gen3X16 bus that provides an effective bandwidth of 12.5GB/s.
GPU
GPUs are designed to generate polygon-based computer graphics. In the recent years, due to the need for realism in recent computer games and graphic engines, GPUs have accumulated large processing powers. A GPU is a parallel programming setup involving GPUs and CPUs that can process and analyze data in a similar way as an image or any other graphic form. GPUs were created for better and more general graphic processing, but were later found to fit scientific computing well.
Back in 2001, Matrix multiplication was computed on a GPU for the very first time. LU factorization was the first algorithm that was implemented on a GPU in 2005. The main challenge was the lack of high-level language, and the researchers had to comprehend the low-level language to understand the graphics processing.
In 2006, Nvidia came out with a high-level language named ‘CUDA’, which helps write programs for graphic processing in a high-level language. This was probably one of the most significant change in the way researchers interacted with GPUs.
minimum gpu requirements for machine learning
ENVI Deep Learning 1.2 uses TensorFlow version 2.4 and CUDA 11, both of which are included in the installation. System requirements are as follows:
- Base software: ENVI 5.6.1 and the ENVI Deep Learning 1.2 module
- Operating systems:
- Windows 10 (Intel/AMD 64-bit)
- Linux (Intel/AMD 64-bit, kernel 3.10.0 or higher, glibc 2.17 or higher)
- Hardware:
- NVIDIA graphics card with CUDA Compute Capability version 3.5 to 8.6. See the list of CUDA-enabled GPU cards. A minimum of 8 GB of GPU memory is recommended for optimal performance, particularly when training deep learning models.
- NVIDIA GPU driver version 460.x or higher
- A CPU with the Advanced Vector Extensions (AVX) instruction set. In general, any CPU after 2011 will contain this instruction set.
To determine if your system meets the requirements for ENVI Deep Learning, start the Deep Learning Guide Map in the ENVI Toolbox. From the Deep Learning Guide Map menu bar, select Tools > Test Installation and Configuration.

machine learning lab requirements
We’re so excited that you’re interested in learning about machine learning. We know it can be a pretty intimidating subject, but we’re here to help you feel more confident about tackling it.
To start with, make sure you have a computer with Python installed and the Anaconda distribution of Python installed. You can download Python from https://www.python.org/downloads/. To install Anaconda, simply follow the instructions on the linked page.
After that, you’ll want to download this course’s code base (see below) and open it up in Jupyter Notebook (available here: https://jupyter.org/). This will give you access to all of our tutorial material and lab exercises so that you can follow along as you learn!
As far as requirements go, there are a few things that will help make things easier for you as we go through this course together:
-Strong mathematical background (we’ll cover this in depth later on!)
-An understanding of Python basics (you can learn those here: http://learnpythonthehardway.org/)

Hardware requirements for machine learning
The first thing you should determine is what kind of resource does your task requires. Let’s have a look how different tasks will have different hardware requirements:
- If your tasks are small and can fit in a complex sequential processing, you don’t need a big system. You could even skip the GPUs altogether. A CPU such as i7–7500U can train an average of ~115 examples/second. So, if you are planning to work on other ML areas or algorithms, a GPU is not necessary.
- If your task is a bit intensive, and has a manageable data, a reasonably powerful GPU would be a better choice for you. A laptop with a dedicated graphics card of high end should do the work. There are a few high end (and expectedly heavy) laptops like Nvidia GTX 1080 (8 GB VRAM), which can train an average of ~14k examples/second. In addition, you can build your own PC with a reasonable CPU and a powerful GPU, but keep in mind that the CPU must not bottleneck the GPU. For instance, an i7-7500U will work flawlessly with a GTX 1080 GPU.
- If you are working on complex problems or are a company that leverages deep learning, you should probably build your own deep learning system or use a cloud service.
- If your task is of a larger scale than usual, and you have enough money to cover up the cost, you can opt for a GPU cluster and do multi-GPU computing. Also, there are more powerful options available – TPUs and faster FPGAs – which are designed specifically for these purposes.
Furthermore, a GPU can perform convolutional/CNN or recurrent neural networks/RNN based operations. It can also perform operations on a batch of images of 128 or 256 images at once in just a few milliseconds. However, the power consumption is around ~250 W and requires a full PC that additionally requires 150 W of power, which leads to a total of 400W.
Applications like virtual or augmented reality goggles, drones, mobile devices, and small robots do not have this much power. Also, in case of autonomous cars and smart cameras, where live video is necessary, image batching is not possible, as video has to be processed in real-time for timely responses.
In the future, we might see more powerful devices that won’t require that much power and work on mobile platforms/devices.
Conclusion
Machine learning is a promising field and with new researches publishing every day. Today’s AI requires a lot of resources to train and produce accurate results. However, there will be more human friendly AI systems in the near future.
eInfochips offers artificial intelligence and machine learning services for enterprises to build customized solutions that run on advanced machine learning algorithms. With more than two decades of experience in hardware design, we have the understanding of hardware requirements for machine learning. If you are planning to implement machine learning for your business and are in search of customized hardware, please get in touch with us.